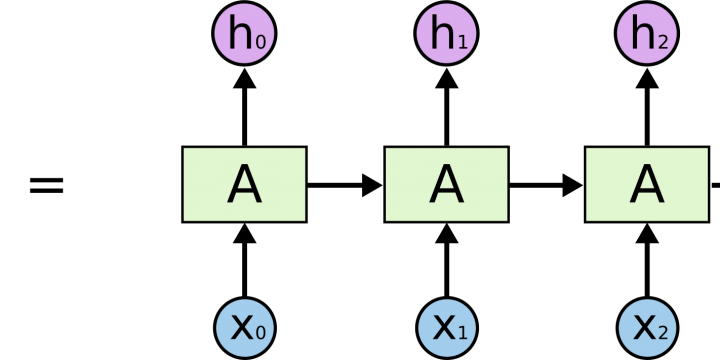
Exploring the boundary between accuracy and performances in recurrent neural networks
When it comes to interpreting streams of data using modern artificial intelligence techniques, such as audio in speech recognition, computational requirements of state-of- the-art models can easily skyrocket and result in huge power requirements. However, accepting a small loss in their accuracy can go a long way in reducing their resource impact. This work explores the boundary between accuracy and performances in such a context. By Alessandro Pappalardo PhD student @Politecnico di Milano Modern artificial intelligence approaches to problems such as image captioning, i.e. describing content of an image, can already have significant computational requirements. On top of that, scaling such techniques from processing a single data point, e.g. an image, to a sequence of them, e.g. a video, increases their requirements non-linearly. The reason is that interpreting a…