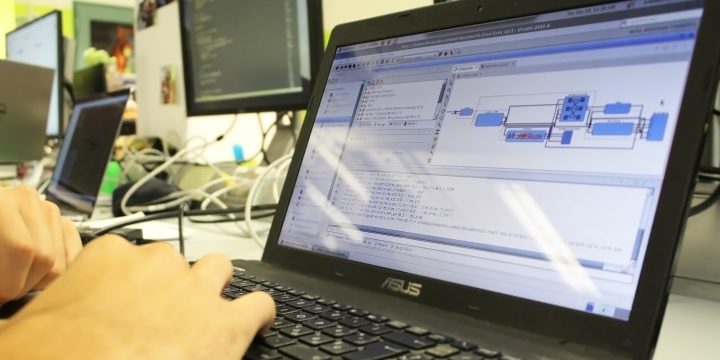
Accelerating Machine Learning: the hard case of generic models
Following the spreading of applications powered by Machine Learning models, the issues arised on how to engineer their development and deployment. This is particularly problematic when the applications should run under stringent performance requirements and in a complex environment like a cloud infrastructure with diverse hardware resources (CPUs, FPGAs, etc.). We performed initial work on this issue and proposed an approach that achieved a 3x speedup over the common case, suggesting practices that pave the way for developing more systematic guidelines and tools. By Alberto Scolari PhD Student @ Politecnico di Milano, working on reconfigurable computing systems at NECSTLab Operational-izing ML: system issues Machine Learning (ML) models are spreading inside companies, as a basis for their business. Nonetheless, applying ML to your business requires theoretical and technical efforts. As willing-to-be system architects,…